What Is The Data Science Process?
5 Mins Read
Published on: 08 December 2023
Last Updated on: 09 September 2024
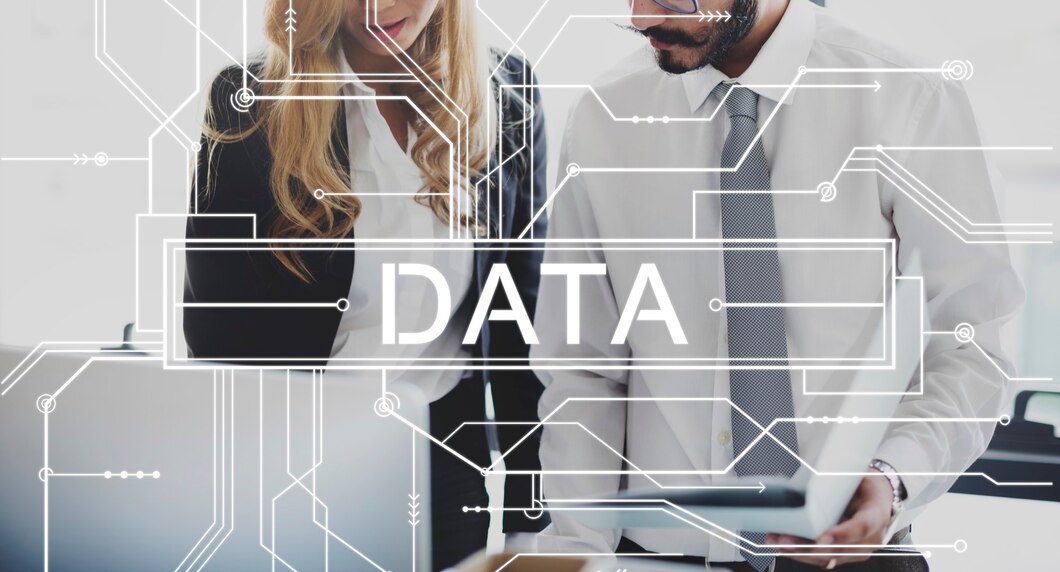
toc impalement
Although data scientists may hold differing opinions on the interpretations of a particular dataset, there is widespread consensus among data science professionals regarding the importance of adhering to the data science process. This structured framework serves as a guide to navigate through a data science project. Various frameworks exist, some tailored for business applications and others optimized for research scenarios.
This article aims to elucidate the most widely adopted data science process frameworks, identifying the most suitable for specific use cases. Additionally, it will delve into the core components that constitute each framework.
A data science course can immensely benefit learning the data science process by providing structured guidance, hands-on experience, and a comprehensive understanding of the key concepts and tools involved. One of the best ways to learn data science is by undergoing a dedicated data science course. Imagine you get a chance to learn data science in IIT Madras! The data science course offered at IIT Madras typically follows a well-designed curriculum that introduces learners to the fundamental concepts of data science, including data collection, cleaning, exploration, modeling, and interpretation. This structured approach helps learners grasp the sequential steps involved in the data science process.
Now that you know how to learn the data science process, let’s delve into understanding what is data science and the data science process.
What Is Data Science?
Data Science is an evolving field whose significance continues to amplify daily. It is the latest buzzword in information technology, and its market demand is on a consistent upward trajectory. The escalating need for organizations to translate data into actionable insights fuels the burgeoning demand for Data Scientists. Major industry players like Google, Amazon, Microsoft, and Apple have emerged as prominent recruiters in this field. Notably, Data Science has become an increasingly coveted domain for IT professionals.
A recent report by Precedence Research, the demand for Data Science is projected to experience a Compound Annual Growth Rate (CAGR) of 16.43%, reaching a substantial market value of 378.7 Billion over the forecast period from 2022 to 2030.
Data Science is an multidisciplinary field that blends mathematics, statistics, machine learning, and computer science elements. Its core involves collecting, analysing, and interpreting data to extract valuable insights, aiding decision-makers in making well-informed choices.
In today’s diverse industries, Data Science is pivotal in predicting customer behavior, identifying trends, and uncovering new opportunities. Businesses leverage it for informed decision-making in product development and marketing. Furthermore, it is a powerful tool for fraud detection and process optimization. Governments also harness Data Science’s capabilities to enhance public service delivery efficiency. Data Science simplifies data analysis, extracting meaningful insights by integrating statistical and mathematical principles, programming proficiency, and domain expertise.
Data Science Process/ Data Science Lifecycle
The data science process is a methodical approach to addressing a data-related challenge. It offers a systematic framework for formulating your problem as a question, determining the most effective solution, and subsequently presenting the resolved issue to stakeholders.
The data science process is also known as the data science life cycle. These terms are interchangeable, referring to a workflow process commencing with data collection and deploying a model to address specific questions. The key steps in this process include:
The life cycle of a Data Science project involves essential steps that ensure a meticulous and precise approach:
Problem Identification: The cornerstone of any Data Science venture, this stage involves understanding the utility of Data Science in the domain, with domain specialists and data scientists collaborating to identify challenges and solutions.
Business Understanding: Business goals are formulated based on customer needs, ranging from predictions and sales boosts to loss minimization and process optimization.
Collecting Data: A foundational phase where data collection is the bedrock for achieving business objectives. Information from surveys, software systems, historical archives, and daily transactions is harnessed for a comprehensive understanding.
Pre-Processing Data: Large volumes of data are extracted from various sources, transformed, and processed into a unified format. A data architect is pivotal in structuring data warehouses and executing Extract, Transform, and Load (ETL) processes.
Analyzing Data: With data ready in the required format, this critical step involves a deep dive into data analysis using statistical tools. A data engineer plays an important role in exploratory data analysis (EDA) to uncover essential features and data distribution.
Data Modelling: Post-analysis, key components are retained, and decisions on how to model the data are made. Machine Learning engineers apply various algorithms to refine the data, often testing models with dummy data.
Model Evaluation/Monitoring: Determining the most effective data modeling method involves testing the model with real-world data. Continuous monitoring is crucial for improvement, especially when dealing with minimal data points.
Model Training: Once the task, model, and data drift analysis are determined, the model undergoes training. Parameters are fine-tuned for accuracy, and during production, the model is exposed to actual data with ongoing monitoring.
Model Deployment: The model is exposed to real-time data, deployed through various channels, marking a critical phase as it encounters the complexities of the real world.
Driving Insights and Generating BI Reports: Post-deployment, the model is utilized to gain insights for strategic decisions linked to business objectives. Reports track progress and process indicators, providing valuable insights.
Taking a Decision Based on Insight: The culmination of the process, where meticulously completed steps lead to reports aiding in crucial decisions. Insights gained from data science contribute to strategic decision-making, such as predicting the need for raw materials benefiting business growth and income production.
Conclusion
The data Science process is a dynamic journey that transforms raw data into actionable insights. Emphasizing problem identification, data collection, analysis, and model deployment it empowers organizations to make informed decisions. The significance of this process resonates strongly in academic institutions, exemplified by IIT Madras, where a robust understanding of Data Science is cultivated. As we navigate the intricacies of this evolving field, the collaboration of academia, industry, and practitioners of data science in IIT Madras ensures a holistic approach to mastering the Data Science process, propelling us toward a future where data-driven insights drive innovation and success.
Read Also:
Comments Are Closed For This Article